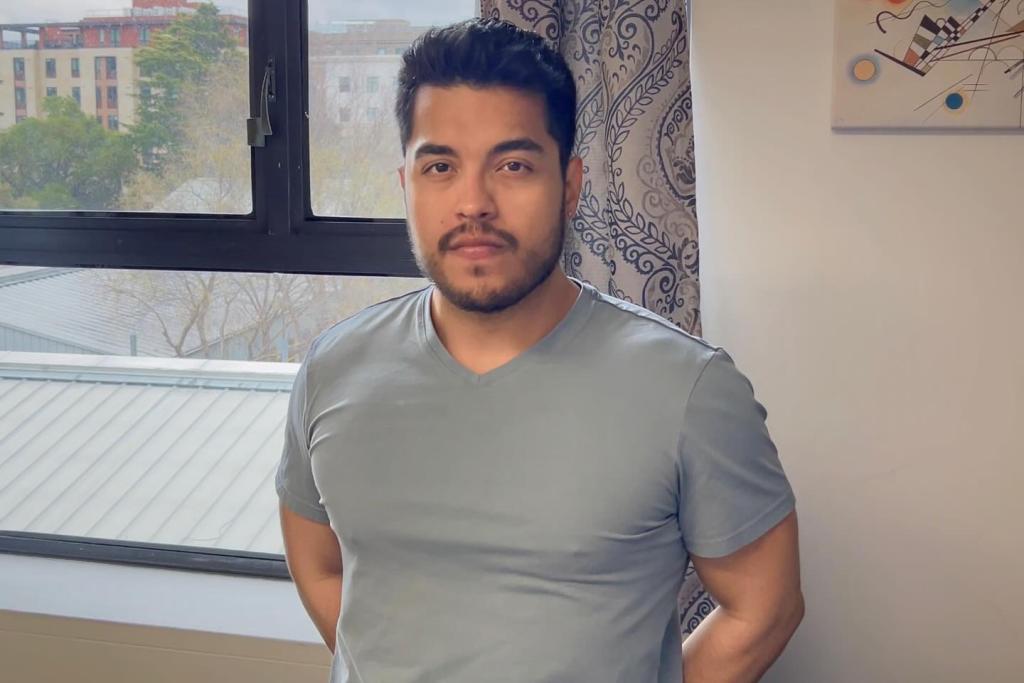
Jose Martin Aveldanes
I am a sixth-year Ph.D. student in Sociology at the University of California, Berkeley. I hold a Senior Data Science Fellow position at the Data Lab (D-Lab) at UC Berkeley. In previous years, I held a fellowship with the National Institutes of Health with the Department of Demography at UC Berkeley, supported by the Eunice Kennedy Shriver Branch for Child and Human Development.
I earned my Bachelor of Science in Sociology from the University of South Carolina, where I received training in quantitative methodology. During my time at South Carolina, I co-authored an article with Drs. Carla Pfeffer and Jennifer Augustine on the amount of time same-sex couples with children spend in activities related to health and well-being.
As an economic sociologist in training, I am broadly interested in market politics, market building, and the use of machine learning tools (ML) and artificial intelligence (AI) in economic action. More specifically, I am curious about the the various strategies market actors use to reduce uncertainty in market transactions. I employ nationally representative longitudinal data, methods of causal inference, and computational social science to investigate these topics.
I have spent the bulk of my graduate career developing a robust set of statistical and computational skills. In 2020, I contributed to the CenSoc project at the Department of Demography at UC Berkeley, involving the linkage of the 1940 Census to files from the Social Security Administration. Additionally, I mentored students within the Cal-ADAR program, guiding them in using R and data science to engage in quantitative social science research. Furthermore, I have taught courses in Data Science at the Advanced Talent Development Program (ATDP), both domestically and internationally, covering subjects such as Machine Learning, Artificial Intelligence, and ethics.
In 2023, I became a Data Science Fellow at the Social Sciences Data Lab (D-Lab) at UC Berkeley. In 2024, I won the Outstanding Graduate Student Instructor Award in the Department of Sociology. My commitment involves collaborating with students, researchers, and faculty to integrate methodologies from quantitative social science and machine learning science, exploring innovative approaches to study economic sociology. I actively participate in mentoring through programs like ATDP and Cal-ADAR, which focus on guiding advanced students at UC Berkeley in their academic pursuits.